Predictive maintenance in aviation is an approach that uses data analysis and artificial intelligence to predict potential failures of aircraft equipment and systems before they occur. This enables airlines and aircraft operators to plan repairs and maintenance proactively, rather than reacting after failures occur.
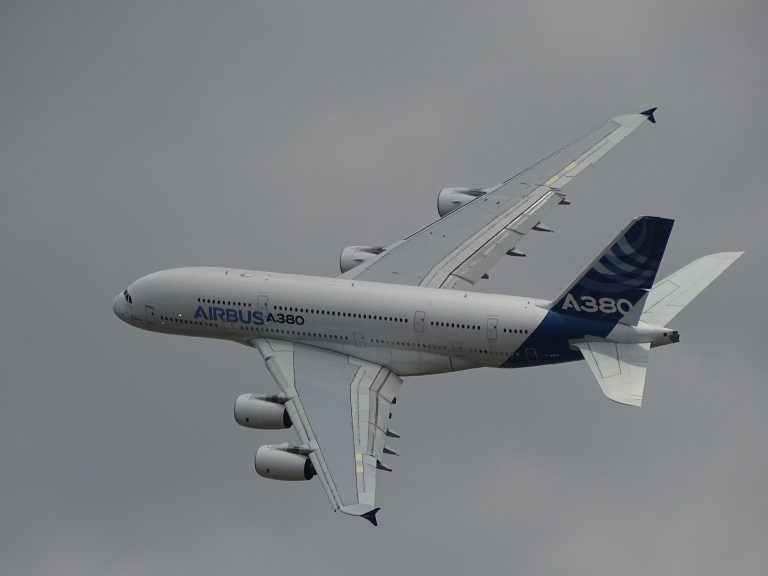
Predictive maintenance – Digital twins
Context & Issue
Challenges
01
Improved fleet availability
02
Reduced maintenance costs
03
Longer system lifetimes
Key Development Points
The right sensor in the right place
- Extensive sensor database for measuring various physical parameters: acceleration, displacement, pressure, acoustics, rotation speed, deformation, temperature, current, etc.
- Analog and digital sensors (MEMS)
- On-board or ground instrumentation (endurance or performance benches).
From on-board sensor to virtual sensor via the digital twin
- On-board instrumentation (anemometer, altitude, temperature, incidence, yaw)
- Test-specific instrumentation: pressures, gauges, anemometer
- Load characterization
- Response characterization: virtual sensor = maximum stress
Difficulty: sensors should preferably be integrated at the design stage. For example, characterization of a dynamic transfer between a bearing defect (spalling) and an acceleration signal detected by the sensor, or a crack at the root of a gear tooth.
A system tailored to your needs
Hardware selection based on:
- Host site: on-board or ground-based,
- Type of storage or transfer,
- Conditioners required for sensors,
- Sampling frequency,
- in-situ processing possibilities,
- Number of measurement channels,
- Type of processing required.
- Possibility of working with hardware already installed.
- Recording triggered by operating parameters, incidents or continuously.
A physical interpretation of the information provided by dynamic static signals is required
The amount of data to be analyzed is very large. A step of information contraction is necessary. The physical interpretation of the indicators enables us to target/select those that are relevant to the analysis of an organ’s state of health.
Finally, a Principal Component Analysis (PCA) is used to reduce the data. The synthesis of the information provided by all the selected indicators can be represented in the form of a global indicator.
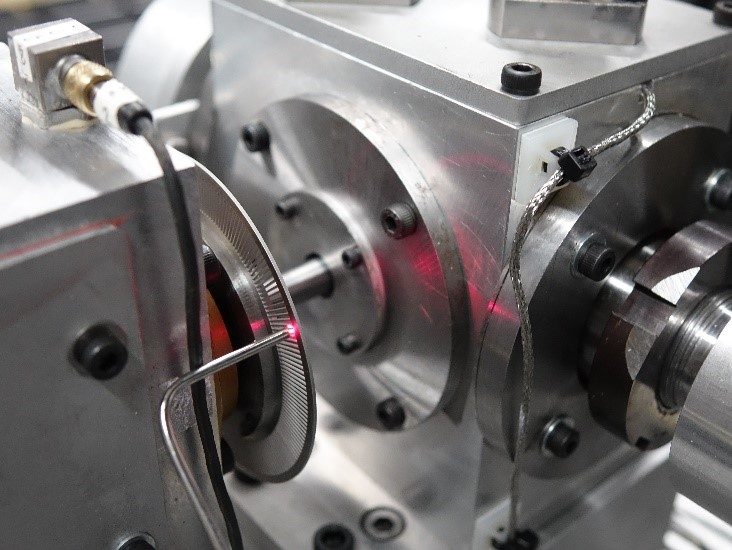
Results and Benefits
Following detection of an anomaly, calculation of residual service life
- Feedback: field feedback / endurance bench testing of components
- Simulation: damage calculation / digital twin (sensor signal/damage transfer)
Decision-making
- Validation of whether or not to replace the component during the next maintenance campaign
- Planning of an urgent maintenance task to replace the faulty component